Data Mining and Knowledge Discovery, 38(5), pp. 2942-2974, 2024
Explainable Artificial Intelligence
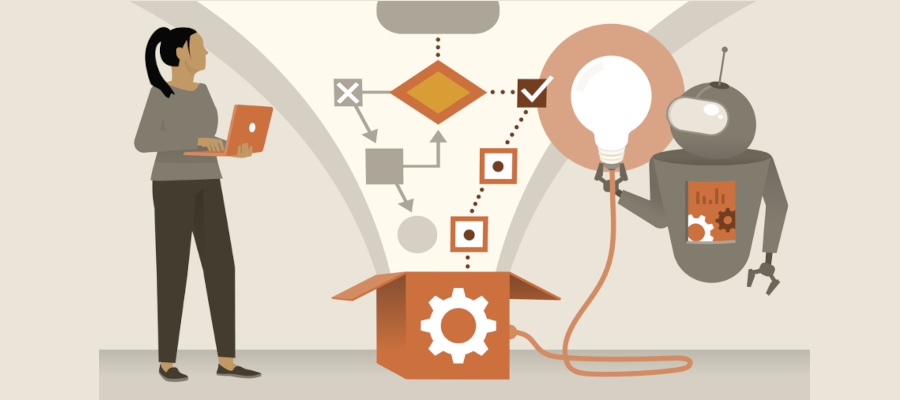
Abstract
As Artificial Intelligence (AI) systems become more complex, there is a necessity to understand their functioning and decisions. To approach this, Explainable AI (XAI) plays an essential role by developing machine learning models that are inherently explainable or interpretable and, when it is not possible, by developing methods to explain these models in a human-understandable way. Visual systems incorporating explainability methods can assist final users and experts in better analyzing the object of interest when it involves the application of highly complex machine learning models. In this project, we aim to develop XAI methods and frameworks to improve the explainability of AI models. One front is the development of image-based-model explainability methods such as Style Augmentation in image classification. Another front is the improvement of the plausibility of text classification explanations through the incorporation of human annotations. We are also interested in advancing methods of attributing computer vision model decisions to images from its training data. Finally, the existing and these new findings are being applied in visualization workflows to improve the degree to which the final user, usually an expert, comprehends the inner workings of the models and the data being analyzed, with a particular focus on the legal domain.
Researchers
Publications
International Conference on Computer Vision Theory and Applications, 2023
Applied Sciences, 12(12), 2022